|
- Info
Faculty in Hunter's Computer Science Department
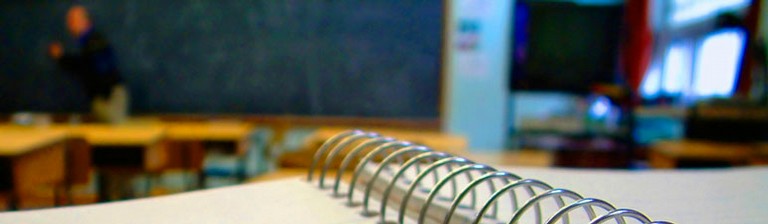
Teaching Computer Science
Spend some time getting to know our professors and you'll discover why Hunter's computer science students thrive before and after graduation:
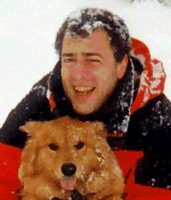
|
William Sakas, Associate Professor, Department Chair
Office: Hunter North 1008-B Email: sakas@hunter.cuny.edu Phone: 212-772-5211 Fax: 212-772-5219 Home Page: https://www.cs.hunter.cuny.edu/~sakas/ Area of Specialty: Computational modeling of human thought processes (in particular natural language), artificial intelligence, machine learning; I'm especially interested in modeling how children learn language. Educational Background: AB from Harvard (Economics), PhD from CUNY (Computer Science). Member, doctoral faculties of Computer Science and Linguistics, CUNY.
|
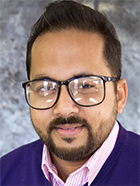 |
Saptarshi Debroy, Assistant Professor
Office: Hunter North 1090-I Email: saptarshi.debroy@hunter.cuny.edu Phone: 212-650-3989 Fax: 212-772-5219 Home Page: http://www.cs.hunter.cuny.edu/~S.Debroy99/ Research areas of particular interest: Computer Networking, Network security, Big Data Networking, Distributed & Cloud Computing, Wireless Networking, Dynamic Spectrum Access, Network Measurements, Internet of Things. Educational Background: BTech in Information Technology from West Bengal University of Technology, India; MTech in Distributed & Mobile Computing from Jadavpur University, India; PhD in Computer Engineering from University of Central Florida, USA.
|
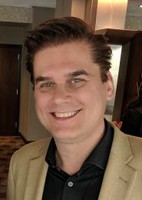 |
Sven Dietrich, Professor
Office: Hunter North 1009 Email:spock@hunter.cuny.edu Phone:TBD Fax: 212-772-5219 Home Page:http://compsci.hunter.cuny.edu/~spock
Research areas of particular interest: computer and network security (denial of service and malware (DDoS, botnets)), cryptography, anonymity, and privacy Educational Background: B.S. in Computer Science and Mathematics, Adelphi University. M.S. in Mathematics, Adelphi University. PhD in Mathematics (with some significant work in Computer Science), Adelphi University
|
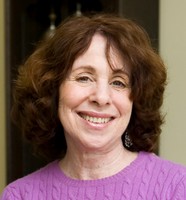
|
Susan L. Epstein, Professor
Office: Hunter North 1090-C Email: susan.epstein@hunter.cuny.edu Phone: 212-772-5210 Fax: 212-772-5219 Home Page: http://www.cs.hunter.cuny.edu/~epstein Research areas of particular interest: problem solving, cognitive modeling, machine learning, and knowledge representation with applications to constraint satisfaction programming, protein folding, two-dimensional layout design, and game playing. Educational Background: B.A. in mathematics from Smith College, M.S. in mathematicsfrom Courant Institute, M.S. in Computer Science from Rutgers, New York University, and Ph.D. from Rutgers.
|
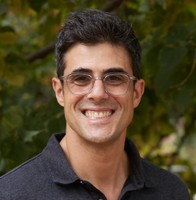
|
Raffi Khatchadourian, Associate Professor
Office: Hunter North 1090-H Email:raffi.khatchadourian@hunter.cuny.edu Phone:212-650-3988 Fax: 212-772-5219 Home Page: https://www.cs.hunter.cuny.edu/~Raffi.Khatchadourian99 Areas of Specialty: Automated software evolution, secure software engineering, refactoring, program analysis, object-oriented programming, aspect-oriented programming, source code recommendation systems Educational background: BS. in Computer Science from Monmouth University, MS and PhD in Computer Science from Ohio State University
|
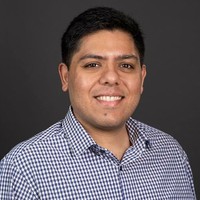 |
Raj Korpan, Assistant Professor
Office: Hunter North 523-B Email: raj.korpan@hunter.cuny.edu Phone: N/A Fax: 212-772-5219 Home Page: https://opencuny.org/rajkorpan/ Areas of Specialty: Artificial Intelligence, human-robot interaction, robot navigation, explainable AI, cognitive modeling, machine learning Educational background: B.S. in Economics & Finance, Bentley University. M.S. in Statistics, Baruch College, CUNY. Ph.D. in Computer Science, Graduate Center, CUNY
|
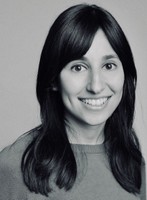 |
Sarah Levitan, Assistant Professor
Office: Hunter North 520-H Email:sarah.levitan@hunter.cuny.edu Phone:TBD Fax: 212-772-5219 Home Page: http://www.cs.hunter.cuny.edu/~slevitan/
Areas of Specialty: spoken language processing, natural language processing, learning speaker states and traits from speech/language Educational Background: BS in Computer Science from Brooklyn College, MS and PhD in Computer Science from Columbia University
|
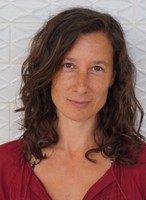 |
Tiziana Ligorio, Doctoral Lecturer
Office:Hunter North 1001-K Email: tligorio@hunter.cuny.edu Phone:212-396-6123 Fax: 212-772-5219 Home Page: https://tligorio.github.io/ Areas of Specialty: Machine Learning, Spoken Dialogue Systems, Constraint Satisfaction Educational background: Ph.D., The Graduate Center of The City University of New York
|
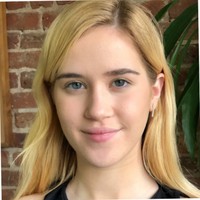 |
Melissa Lynch, Lecturer
Office: Hunter North 1001-K Email: melissa.lynch@hunter.cuny.edu Phone: --- Areas of Specialty: Natural language processing, full-stack web development Educational background: B.A. in Linguistics from the University of Illinois at Urbana-Champaign; M.A. in Computer Science from Hunter College, City University of New York
|
 |
Gennadiy Maryash, Lecturer
Office:Hunter North 1047 Email: gmaryash@hunter.cuny.edu Phone:212-772-4283 Fax: 212-772-5219 Home Page: maryash.github.io Areas of Specialty: Educational methodology and scalable teaching practices for large lecture, hybrid, and online courses; industry aligned curriculum implementation. Educational background: BA (Philosophy) from Baruch College CUNY, MS (Computer Science) from Lehman College CUNY.
|

|
Saad Mneimneh, Assistant Professor
Office:Hunter North 1090-L Email: saad@hunter.cuny.edu Phone: 212-772-5209 Fax: 212-772-5219 Home Page: http://www.cs.hunter.cuny.edu/~saad/ Area of Specialty: Application of mathematical modeling and algorithmic techniques to scheduling, load balancing, and more recently computational biology. Educational Background: Ph.D., Computer Science, Massachusetts Institute of Technology
|
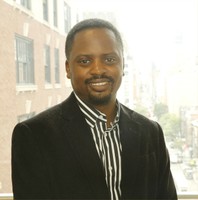 |
Oyewole Oyekoya, Associate Professor
Office:Hunter North 1001-T Email: oo700@hunter.cuny.edu Phone:TBD Fax: 212-772-5219 Home Page: https://wolex.com/ Area of Specialty:visualization, virtual reality, eye tracking, and visual perception. Educational Background:Ph.D., University College London
|
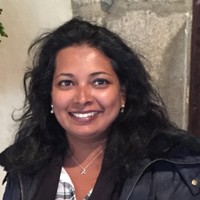 |
Anita Raja, Professor
Office:Hunter North 1090-G Email: anita.raja@hunter.cuny.edu Phone:212-650-5031 Fax: 212-772-5219 Home Page: https://anraja.commons.gc.cuny.edu/ Area of Specialty: artificial intelligence, multiagent systems, metacognition, machine learning, bounded rationality, complex networks, game theory. Educational Background: B.S. in Computer Science with a minor in Math from Temple University, M.S. and Ph.D in Computer Science from UMass Amherst.
|
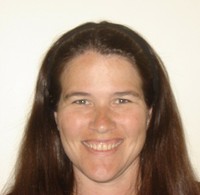 |
Katherine St. John, Professor
Office: Hunter North 1090-D Email: katherine.stjohn@hunter.cuny.edu Phone: 212-772-5368 Fax: 212-772-5219 Home Page: https://stjohn.github.io Area of Specialty: Computaional Biology, Random Structures and Algorithms. Educational Background: A.B., Smith College, M.A., Johns Hopkins University, Ph.D., University of California at Los Angeles
|
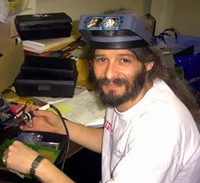
|
Eric Schweitzer, Lecturer
Office: Hunter North 1000-E Email: eric.schweitzer@hunter.cuny.edu Phone: 212-772-4349 Fax: 212-772-5219 Area of Specialty: Theory of computation, logics of knowledge and probablistic reasoning. Educational Background: BS and MA from SUNY Stony Brook
|
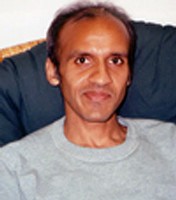
|
Subash Shankar, Associate Professor
Office: Hunter North 1000-F Email: subash.shankar@hunter.cuny.edu Phone: 212-650-3992 Fax: 212-772-5219 Home Page: http://www.cs.hunter.cuny.edu/~sshankar Area of Specialty: Formal Methods, Software Engineering & Programming Languages, Artifical Intelligence Educational Background: BS in Electrical Engineering from Virginia Tech; MS in Computer Science from Rensselaer Polytechnic Institute; MS in Mathematics from University of Minnesota; PhD in Computer Science from Univ. of Minnesota
|
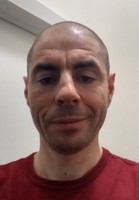 |
Pavel Shostak, Doctoral Lecturer
Office:Hunter North 520-F Email: ps57@hunter.cuny.edu Phone: TBD Fax: 212-772-5219 Education: PhD in Computer Science from Graduate Center of CUNY, MS in Mathematics from Lomonosov's Moscow State University. Areas of Specialty: Cryptography, Group Theory.
|
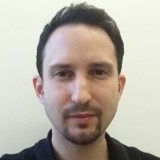 |
Justin Tojeira, Lecturer
Office:Hunter North 1008-C Email: justin.tojeira@hunter.cuny.edu Phone: TBD Fax: 212-772-5219 Education: MS in Computer Science from Graduate Center of CUNY, BS in Computer Science from Brooklyn College.
|

|
Ioannis Stamos, Professor
Office: Hunter North 1090-K Email: istamos@hunter.cuny.edu Phone: 212-772-5215 Fax: 212-772-5219 Home Page: http://www.cs.hunter.cuny.edu/~ioannis/ Area of Specialty: Computer Vision with an emphasis on 3-D modeling using range data, 3-D Visualization, Range and Image Sensor Fusion, Sensor Planning. Educational Background: Diploma of Engineering from the Department of Computer Engineering and Informatics, University of Patras, Greece. M.S. in Computer Science, M.Phil., and Ph.D, from Columbia University.
|
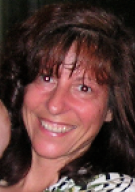
|
Felisa Vázquez-Abad, Professor
Office: Hunter North, 1000-B Email: felisav@hunter.cuny.edu Phone: 212-772-5634 Fax: 212-772-5219 Homepage: Under Construction Area of Speciality: Adaptive control of stochastic discrete event process, simulation, theory and applications of automatic learning for optimization, queueing networks, weak convergence theory, probability theory and Markov decision processes. Educational Background: Ph.D. Brown University, Applied Mathematics.
|
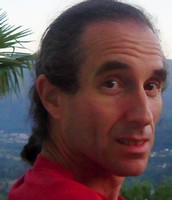
|
Stewart Weiss, Associate Professor
Office: Hunter North 1090-J Email: stewart.weiss@hunter.cuny.edu Phone: 212-772-5469 Fax: 212-772-5219 Home Page: http://www.compsci.hunter.cuny.edu/~sweiss Area of Specialty: Software Quality Assurance theory and Methods. Educational Background: PhD in Computer Science, Courant Institute of Mathematical Sciences, New York University.
|
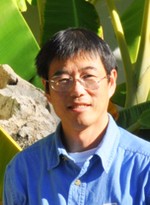
|
Lei Xie, Professor
Office: Hunter North, 1000-H Email: lxi0003@hunter.cuny.edu Phone:858-822-3686 Fax: 858-822-0873 Home Page: http://compsci.hunter.cuny.edu/~leixie/ Area of Specialty: bioinformatics, systems biology, molecular modelling and simulation. Educational Background: B.S. in Polymer Physics from University of Science and Technology of China; M.S. in Computer Science from Rutgers University - New Brunswick; Ph.D. in Chemistry from Rutgers University - New Brunswick.
|
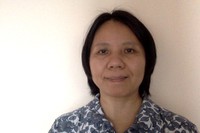 |
Tong Yi, Doctoral Lecturer
Office: Hunter North 1001-L Emaill: ty680@hunter.cuny.edu Phone:TBD Fax: 212-772-5219 Homepage: TBD Areas of Interest:Computer Science Education Education: PhD in Computer Science, Louisiana State University
|
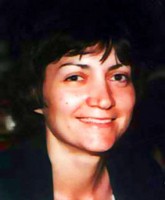 |
Christina Zamfirescu, Professor
Office:Hunter North 1008-C Email: christina.zamfirescu@hunter.cuny.edu Phone:212-650-3854 Fax: 212-772-5219 Area of Specialty:Graph Theory: algorithmic combinatorics & networks, molecular complexity, intersection digraph representation. Educational Background:Master's in O.R. University of Bucharest, Ph.D. in Natural Sciences (Graph Theory /Computer Science)- RWTH-Aachen, Germany.
|
|
|
Affiliated Faculty
|
|
|
|
|
|
Emeritus Faculty
|
|
 |
Daniel Cohen, Professor Emeritus
Area of Specialty: Graph Theory, Algorithms, Combinatorics, Computer Law Educational Background: BS in Mathematics from Princeton, Ph.D. in Mathematics from Harvard University, J.D. from Columbia University
|
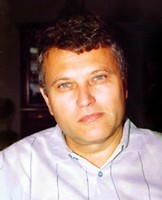 |
Constantin Negoita, Professor Emeritus
Area of Specialty: Computer Logic, Fuzzy Sets and Systems. Educational Background: Ph.D. in Information Science from the University of Bucharest
|
 |
Cullen Schaffer, Professor Emeritus
Area of Specialty:Computation-intensive approaches to data analysis. Educational Background: BA from Harvard, PhD from Rutgers.
|
 |
Virginia Teller, Professor Emeritus
Area of Specialty: Natural Language Processing, Artificial Intelligence Educational Background: BA in French Literature from Cornell University, MA and Ph.D. in Linguistics from New York University.
|
NEXT: Part Time Computer Science Faculty
|
-
Prof. Sarah Ita Levitan Secures Google Research Award in Cyber Security!
Oct 26, 2023
-
Prof Stamos and PhD Student Paper Accepted to IROS 2023!
Sep 26, 2023
-
CSCI PhD Candidate Presented Paper at ASE 23!
Sep 26, 2023
-
Prof. Debroy Wins Google Research Award in Cybersecurity!
Sep 26, 2023
-
Interdisciplinary team receives $20MM from NSF to develop AI Institute, ARNI; Professor Sarah Ita Levitan will be senior personnel on the grant
May 19, 2023
-
Isabel Stec earns John P. McNulty Scholarship
Apr 24, 2023
-
Yiming Tang to commence tenure-track Assistant Professorship at RIT
Apr 24, 2023
-
Professor Anita Raja receives CUNY Research Planning Grant
Mar 17, 2023
-
Hunter Students attend MIT's Quantitative Methods Workshop
Mar 06, 2023
-
Alumni Interview Series: Sarah Mathew – Graduate Student and Research Assistant, Georgia Tech
Jan 30, 2023
|